Learning:
Finding Stories in Census Data
Emily Alpert Reyes on how to find promising needles in Census haystacks
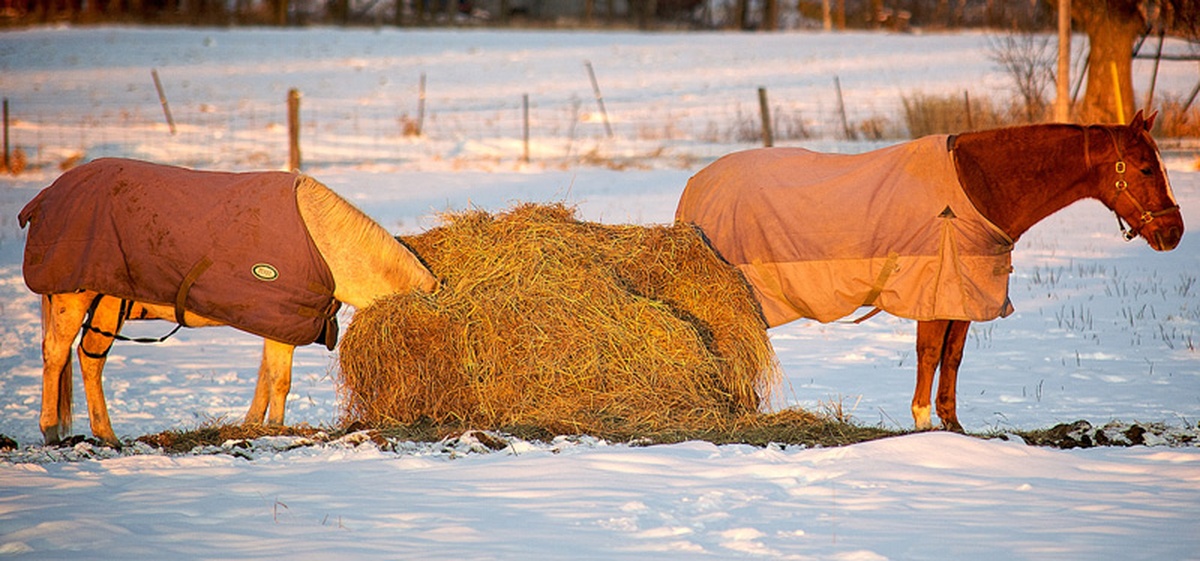
It’s much easier than this. (Perry McKenna)
Once you’ve learned how to pull data from the Census website, the next step is detecting stories in the data. After all, your editors don’t want data from you—they want stories.
Whenever I approach a new dataset, these are two simple things I look for to start generating story ideas: trends and outliers. Getting from the data to the story will be different every time you dig into the numbers, but this method can be a useful way of starting your search.
Outliers
Outliers are easy to find—and they can be truly fascinating stories. What places in your area have the lowest levels of Internet use? The most single parents? The most multiracial people? If the answers end up surprising you, your readers will probably also be interested—and want to know the reasons behind those surprising facts. It’s a great hook for traditional reporting—getting out and knocking on doors and finding the real people who are living those statistics.
One simple way to find outliers is to search for places that fall at the extremes: the cities, counties or even census tracts that are at the highest or lowest end when it comes to something you find interesting. To start seeking out outliers, download a table that interests you from American FactFinder, including a long list of geographic locations. For instance, you could compare bicycle commuting in all counties in the United States, part time workers in all census tracts in your city, or military dependents in all cities in your state. Once you’ve downloaded the table into Excel, use filters to sort the data from highest to lowest or vice versa.
If you cover a particular city, for instance, you could pull a table of all metropolitan areas in the United States and then sort the data to see if the city that you cover falls near the bottom or the top for a particular indicator you’re interested in. (To make it easy on yourself, highlight the row for your city in bright yellow or green in Excel.)
You can also pull tables for “All Places”—cities and unincorporated areas—but keep in mind that comparing tiny towns to huge cities can be a bit deceptive. When I was on the demographics beat at the LA Times, I frequently found that a cluster of small cities south of Los Angeles seemed to be at the extremes—for instance, having higher poverty or rates of single motherhood than Los Angeles—but that masked the fact that there were areas of comparable size within Los Angeles that were actually more extreme. If you write about a large city, you’ll want to be cautious in using “All Places” to detect trends in your region for this reason.
One of my favorite stories on the demographics beat, “L.A. and Orange counties are an epicenter of overcrowded housing,” was born out of this strategy. The ACS includes data about “occupants per room,” the number of people per room in a housing unit. I used the data to calculate the percentage of housing units that were considered “crowded” by government standards, then ranked places by their crowding rate.
I originally downloaded the table for all places in the United States and noticed that several areas south of Los Angeles shot to the top of the crowding list. With the help of our data and graphics team, I was able to pull the same data for zip codes and map it, revealing that some areas within the city of Los Angeles had a higher percentage of crowded homes than the towns that initially grabbed my attention. In general, we saw a constellation of local cities and areas that were unusually crowded compared to the national average.
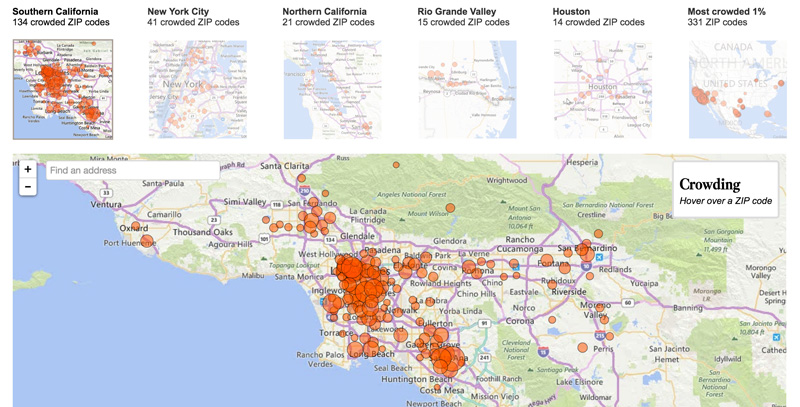
Screenshot of the LA Times interactive “Mapping the country’s crowded homes.”
A simple ranking, however, can also be misleading. We noticed that a scattering of extremely small zip codes in Alaska had very high rates of crowded housing, but they had so few people that their crowdedness wasn’t as noteworthy. My coworker Ryan Menezes used a statistical method to better compare census tracts large and small.
As Ryan explains it, “the calculation captured how much each tract’s crowding rate deviated from the national average…and scaled it appropriately based on the total number of homes in the area. Doing this allowed us to see, for example, that a crowding rate of 47% in a highly populated Santa Ana tract was more severe than 85% of homes being crowded somewhere in rural Alaska.” We explained this adjustment in our methodological notes to the story.
The story about crowded housing used 5-year-estimates from the American Community Survey—data gathered over the course of five years—to get the most accurate estimates possible for small areas such as zip codes. This meant that we couldn’t make sweeping claims about whether crowding had changed during the recession. But since our focus was why Southern California was unusually crowded, that was OK—the point for us was what made this phenomenon more extreme in and around Los Angeles, not how it had changed over time.
Another important thing to keep in mind is that the smaller the area you want to zero in on, the less you can pinpoint what’s happening in a specific year. The reason: if you’re using ACS data, a very small area has so little data gathered in a single year that it’s less reliable, and the margins of error become so large that the data can be difficult to trust. You’ll want to use 5-year-estimates for very small areas such as census tracts.
As I mentioned before, the great thing about finding an outlier is that it points you right to where you need to do your shoe-leather-reporting. When I went looking for families living in crowded conditions, I knew which neighborhoods to go to and which community groups to check with. With some work, I identified a family of seven who were sharing a studio apartment, and living in one of the neighborhoods that topped our list for crowded housing in Los Angeles.
Another one of my stories, “L.A.’s close-knit Tongan community struggles with poverty,” used this same strategy—but from a slightly different approach.
I got tipped off to an intriguing outlier by a local group, Asian Americans Advancing Justice, which was trying to debunk the “model minority” stereotype for Asians and Pacific Islanders. It issued a report pointing out that some Asian and Pacific Islander subgroups were faring much worse than others. For instance, in Los Angeles, people of Tongan descent had staggeringly high poverty rates, the group pointed out in its report.
In this case, I was looking for groups that were outliers—not places. Using American Factfinder, I clicked “Race or Ethnic Groups,” then the tab for “Detailed Groups.” This allows you to see data not just for racial groups, but for people who trace their roots to a particular country or ethnic group. I selected all ancestry groups and all racial groups, then searched for tables on poverty. The most recent data broken down by such small groups are from the decennial Census—the 2010 ACS 5-year Selected Population Tables. I narrowed my search to the Los Angeles metropolitan area, then downloaded the information to compare how Tongan Angelenos were faring compared to other groups.
Because there are relatively few people of Tongan descent in Los Angeles, the margins of error for their poverty rates, educational levels, and other indicators were very broad. I had to use data spanning a long period to ensure the greatest accuracy possible, and I had to be cautious about my claims because of the large margin of error. Still, even with a hefty margin of error, Tongan Angelenos stood out as being unusually likely to live in poverty and have other disadvantages, including low educational attainment. You could pull this same kind of data to look at the statistics for ethnic subgroups in your area—say, Armenian Americans, or Iraqi Americans, or Cambodian Americans. Or you could focus on a particular indicator and see how different groups rank. This data can be a terrific source for stories about groups that are quietly struggling—or succeeding—in your area.
Trend Stories
Trend stories are the kind of stories you’re most likely to be pitched from think tanks such as the Pew Research Center and other research groups on the census beat.
To come up with trend stories on my own on the demographics beat, I tried to make a regular practice of picking a new indicator in the ACS each week and pulling tables for the last five years in Los Angeles. This is a little tricky because you’ll need to download and compare multiple tables. I find it helpful to make a new spreadsheet, pull the data for each year and single out the thing I’m interested in—say, the poverty level in Los Angeles County—and then copy that into the new spreadsheet. You could do the same for whatever area or topic you’re interested in. Make it a habit and just keep looking for anything interesting. Keep in mind, too, that sometimes lack of change can also be a story—for example, if a lot of money has been invested into changing something that does not appear to have changed.
Trends are also a natural choice for stories that emerge from new data from the Census Bureau. When the bureau is slated to release new data, I try to prepare in advance by pulling the same indicators for the four previous years. Then when the new data is released, I plug it into my spreadsheet to see if there’s an interesting pattern.
For one story based on of a fresh release of Census Bureau data, I enlisted the help of one of our Los Angeles Times data analysts, Sandra Poindexter, to help me pull unemployment data by age group. The results showed that young adults were much less likely to have work and that their unemployment levels remained stagnant even during the recovery.
Whenever I compare a statistic over time, however, I contact the Census Bureau to make sure that the indicator hasn’t changed in some way over time—for example, that they haven’t changed the way they ask a question. You’ll also want to ask the Census Bureau about exactly what a particular indicator—such as “poverty”—means.
Trend stories can also come out of another data source that I used frequently on the census and demographics beat: the General Social Survey. The GSS is a long-running survey on American life that probes a lot of areas—religion, sex, childrearing—that the Census Bureau just doesn’t touch. It’s a project of the independent research organization NORC at the University of Chicago, and it is woefully underused by journalists, in part because getting into the data is a little less intuitive. Start with this link, then click, “General Social Survey (GSS) Cumulative Datafile 1972-2012 (SDA 4.0) .”
You’ll find a dizzying array of questions on a number of topics here. Unfortunately, not all questions are asked every year. You’ll have to test it out to see when they’re asked, or ask NORC to provide its key to which questions were asked when. I’ve used that a few times to try to narrow down what I want to look at.
For one of my stories, “Fewer men are paying for sex, survey suggests,” I used this data to explore how likely men were to say they had paid someone for sex or been paid for it. The technique I use to track a trend over time is to enter the name of the topic question I want to look at in the “Row” space, and then write YEAR in “Column.” You can also filter the results to look solely at male respondents, for example (Sex(1)) or other subgroups. In this case, because relatively few men answered that question in any one year, I grouped the answers together into similar timespans, to get a more substantial sample. We found that between 1991 and 1996, nearly 17% of men answered yes, compared to only 13.2% between 2006 and 2012.
Using the filters, I was able to identify one possible reason: Men who had served in the military were much more likely to say they’d engaged in paid sex, and military service is growing less common. But paid sex had also declined among veterans, too. The results were surprising to many researchers and advocates I spoke to, providing a provocative jumping-off point for a story about whether, in fact, men were less likely to pay for sex than in the past.
As these examples show, the data are just the beginning. Thinking about outliers and trends are only two ways of coming up with stories—but they’re useful patterns to watch for when you start exploring any new dataset.
Credits
-
Emily Alpert Reyes
Emily Alpert Reyes is a reporter with the Los Angeles Times, currently covering City Hall. Before she joined the Times, Emily worked for the pioneering nonprofit news website voiceofsandiego.org, where she won national honors for her reporting on education. She is a graduate of the University of Chicago.